Data Fabric Meaning
The definition of Data fabric is a software architecture for data management that unifies and integrates data across multiple systems. Data fabric uses a variety of approaches to create a unified data management system that allows organizations to access, process, and share data more efficiently.
Implementing a data fabric helps organizations store and manage data more efficiently and provide better services. Data fabrics are commonly used by companies that have large amounts of diverse types of data and use cases for their applications.
Data fabric integrates and manages multiple types of storage systems -- including file, object, block and cloud storage -- for unified access to structured and unstructured data.
Data fabrics are typically built on a distributed architecture based on nodes that are connected by high-speed networks, such as InfiniBand or Ethernet. The idea is to simplify the integration of disparate storage technologies into one cohesive system that works together to meet an enterprise's needs.
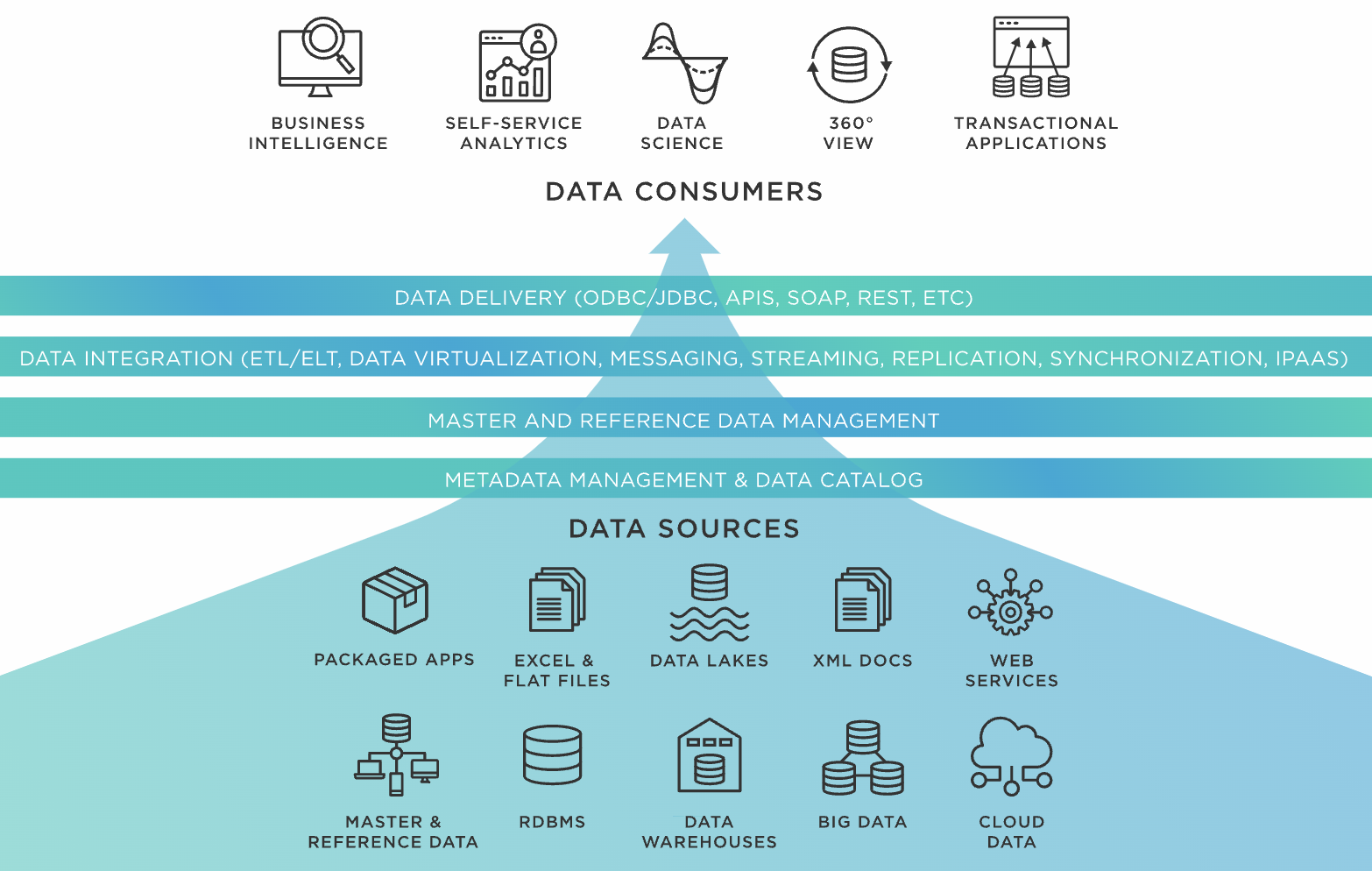
How to measure it?
Data fabric can be built on-premises or in the cloud. It allows organizations to move data where it needs to be accessed most quickly or cost-effectively. This allows users to access data in ways they haven't been able to before. Much of today's data is stored in the cloud rather than on premises. That makes it difficult for organizations to integrate all their data into one system for analysis. Data Fabric solves that problem by aggregating multiple sources of information from both on premises and cloud environments into a single unified platform.
The term "data fabric" is often used interchangeably with "hybrid cloud." Forrester Research defines hybrid cloud as a "cloud computing environment that uses a mix of on-premises, private cloud and third-party, public cloud services with orchestration between these platforms."
Data Fabric has a number of components. It's the new way to think about cloud computing, analytics and data management. The philosophy is that you can store data anywhere and use any technology to analyze it. Unlike traditional approaches, Data Fabric is easy to install, configure and use. It's also much less expensive than traditional approaches.
How is it different from a data mesh?
As defined above, data fabric is a method of connecting collections of data across several tools in a cohesive way. This means that ensuring data integrity and organization, as well as costs of hosting such data is all-inclusive. This is usually done with a single tool or platform that likely hosts these various data sources in the same place.
Data mesh, on the other hand, allows different teams to collect and store data as they see fit, even if that means doing so on separate platforms. However, they must follow certain guidelines and rules that have likely been laid out by data stewards within their organization, or were directed by their data governance council.
How is it different from data silos?
With the rise of the modern data stack along with the ever increasing number of tools for managing and working with data int he market, it can become really easy for data to become siloed. When this happens, there is little visibility or interaction between several sources of data, resulting in unreliable analytics and insights.
The best data fabric implementations solve this problem and result in data that is no longer siloed. This means that a good data fabric not only results in faster, cleaner data, but makes the data that is being accessed reliable and easy to understand.