Top trends in data governance for 2025: Insights from industry leaders
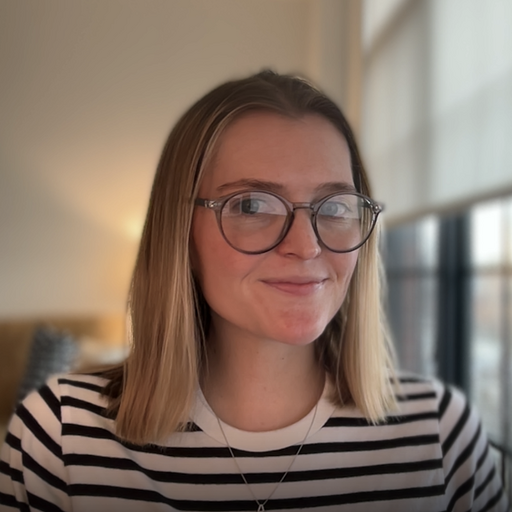
Data governance is rapidly evolving to meet the challenges and opportunities of a data-driven world. Industry professionals predict that 2025 will be a transformative year, with new technologies and strategies reshaping how organizations manage, secure, and leverage their data. This article highlights key trends and forward-looking insights from leaders across industries, providing a glimpse into the future of governance.
The definition of data governance has broadened over the past year, reflecting shifts in priorities and the growing complexity of modern data ecosystems. Professionals are emphasizing:
These shifts demonstrate a focus on enabling innovation and access while upholding security, ethics, and trust.
When asked about the trends and technologies most likely to impact data governance in 2025, professionals pointed to the following areas:
AI and machine learning are transforming data governance by automating critical processes and reducing manual intervention:
As AI matures, it will continue to streamline governance workflows, making them more scalable and effective.
Enhanced data access control features simplify the request and approval process, making it easier for users to get the data they need while staying aligned with organizational policies. These controls encourage a self-service approach, breaking down barriers to data access and increasing adoption across teams.
Dynamic policy management takes this further by automating compliance tasks, such as data anonymization and masking, based on attributes like tags, ownership, or sensitivity levels. This automation reduces manual effort, strengthens data security, and gives users greater confidence in the systems they rely on.
These features are essential in the context of emerging data governance trends, where accessibility, security, and agility are key. By enabling efficient and compliant data access, they support the growing emphasis on democratizing data while maintaining trust and accountability within organizations.
Embedding governance directly into codebases is becoming the standard for organizations seeking scalable and adaptable governance practices. This approach seamlessly integrates governance into modern data workflows, enabling greater efficiency and consistency:
This "governance as code" approach integrates governance directly into agile workflows, making it scalable, consistent, and better suited for quick decision-making. By embedding governance into daily data operations, organizations can confidently and efficiently manage the complexities of modern data ecosystems.
Data is increasingly being treated as a product, with organizations adopting models that emphasize quality, usability, and accessibility. This approach shifts the focus from simply storing and managing data to actively delivering it as a reliable and consumable resource for teams across the organization.
Standardized frameworks are at the core of this model, providing clear benchmarks for data quality, structure, and accessibility. These standards ensure that data consistently meets organizational needs and can be trusted for decision-making and analysis, reducing risks associated with inaccurate or incomplete data.
Collaboration-first approaches underpin the adoption of data-as-a-service models. By breaking down silos and enabling seamless data sharing between teams, these models foster a culture of collaboration. Data consumers, such as analysts and business units, can access the information they need without unnecessary delays, leading to more efficient workflows and better decision-making.
This trend highlights a broader shift toward aligning data strategies with business goals. Treating data as a product encourages data teams to prioritize transparency, reliability, and usability, ensuring they deliver real value. This approach not only enhances internal operations but also opens up opportunities for external data monetization, especially in industries where shared data ecosystems or partnerships drive innovation and revenue.
By adopting data products and data-as-a-service models, organizations are transforming how they manage and utilize data, ensuring it serves as a strategic asset that aligns directly with organizational goals.
Data quality scoring is becoming a significant trend in data governance due to its ability to provide a quantifiable measure of data's reliability and usability. Data quality scoring provides an objective way to assess the quality of data. This is important for organizations to ensure that their data meets certain standards before it is used for decision-making or analysis.
Here’s why it is gaining traction:
Beyond these benefits, data quality scoring directly impacts ROI by reducing costs associated with poor data, such as inaccurate decision-making, inefficiencies, and regulatory penalties. Tools like Secoda enhance this ROI by not only automating the scoring process but also providing actionable insights for improvement. For example, Secoda suggests adding documentation, assigning data owners, or implementing freshness monitors on tables to elevate overall quality. This proactive approach ensures that organizations achieve measurable financial returns while continuously improving their data quality.
Federated governance models provide a balanced way to manage data by combining team autonomy with organizational alignment. In this approach, individual teams have the freedom to govern their own data based on their specific needs and workflows, while still adhering to overarching governance standards set by the organization.
This structure allows teams to operate independently, making decisions and managing data efficiently, while central policies maintain consistency, compliance, and alignment with broader organizational goals. It strikes a balance between flexibility and control, making it especially valuable for large, complex organizations.
A key element of federated governance is the use of data contracts, which formalize expectations for data quality and usage. These contracts promote accountability and collaboration by ensuring all teams operate under a shared framework of governance requirements.
Federated governance offers several benefits. It enhances agility by enabling teams to act quickly without waiting for centralized approvals and improves scalability by accommodating growing data volumes and complexity. By decentralizing responsibilities while keeping centralized oversight, this model allows organizations to innovate and adapt without compromising consistency or compliance.
As organizations seek faster decision-making and more collaborative approaches to data, federated governance is becoming an essential strategy for balancing efficiency and control.
The trends for 2025 highlight the integration of advanced technologies with a stronger focus on accountability and ethical practices. AI-driven automation is reducing governance complexity, while concepts like governance as code and data contracts ensure consistency and reliability. Meanwhile, the growing emphasis on transparency, democratization, and collaboration reflects an understanding that effective governance is not just a technical challenge—it’s a cultural one.
Looking ahead, these trends will have a big impact on industries like healthcare and finance, where regulation and trust are key. In healthcare, data governance will help protect patient privacy while making it easier for hospitals and clinics to securely share data. This kind of collaboration can lead to better patient outcomes. Tools like AI-powered data quality scoring and lineage tracking will ensure that decisions—like diagnoses or treatments—are based on accurate and up-to-date information.
In finance, federated governance models and data contracts will help strike a balance between flexibility and strict compliance. For example, fraud detection teams can operate independently while still following central policies. Semantic layers will also simplify financial data, making it easier for business teams to access and understand complex information without losing sight of regulatory requirements.
These tools and practices can help create smarter, safer ways to work with data—making a real difference in people’s lives and business outcomes. Adopting these practices will be essential for organizations looking to stay ahead in 2025.